There’s an exciting shift happening in the AI domain, and it’s related to the advent of Baby Artificial General Intelligence (BabyAGI). This industry-shaking advancement plans to reconfigure our understanding of AI systems’ capabilities, outgrowing the boundaries set by traditional AI in terms of cognition, decision-making, and continuous learning capabilities. This essay explores and makes clear the importance of BabyAGI in the AI field, dissecting its working mechanism, charting its significance, aligning its contributions to AI development, and speculating the potential challenges and trajectories that could shape the future of AI.
Understanding BabyAGI
Unearthing BabyAGI: A Seminal Leap in the Field of Artificial Intelligence
In the transformative realm of Artificial Intelligence, developments often create ripples in the sea of knowledge that then manifest as waves of discovery. One such wave is currently taking shape, and it’s known as BabyAGI. At its most fundamental, BabyAGI or Baby Artificial General Intelligence is an approach to AI development whose aims are the precise embodiment of a learning-based model of artificial intelligence as opposed to rule-based models.
To truly appreciate the significance of BabyAGI, a context of its contrast against traditional AI must be established. Traditional AI relies on rule-based models, wherein programs operate according to a prescribed set of rules. This approach, albeit beneficial in certain scenarios, often falls short in handling the inherently nuanced and unpredictable nature of real-world interactions. BabyAGI stems from the need to overcome this limitation.
The term “BabyAGI” makes an allusion to the development of a human child, highlighting its principled approach that mimics the way a baby learns and understands the world. Human minds do not follow a defined set of algorithms or rules; rather, they learn from experiences and exposures. Similarly, BabyAGI is aimed at focusing on this learning-based approach, endeavoring to accomplish what is called “Artificial General Intelligence (AGI)”.
Artificial General Intelligence, as differentiated from narrow AI, purports to solve virtually any intellectual task that a human being can. BabyAGI serves as an incubation stage, indicating the infancy of this growing intelligence and its potential to mature into full AGI. It is an ambitious nod at adopting a cumulative learning model, wherein the system learns autonomously, amasses knowledge, applies it to solve problems, and learns further from the results of those actions.
One of the groundbreaking aspects of BabyAGI lies in its “unsupervised learning” strategy. This unique method enables the AI to learn and make sense of data without explicit instruction, augmenting its ability to extrapolate complex concepts from data and to learn continually. It reduces the dependency on extensive labeled data, a critical limitation of current AI techniques such as supervised learning.
The notion of BabyAGI presupposes systems that can understand and interpret the world much like humans, moving away from a narrow mechanical interpretation of situations to a holistic, more nuanced understanding. It corresponds to the development of cognitive abilities and reasoning skills in the AI, thereby achieving a more human-like intelligence.
While the journey to fully actualizing BabyAGI still has a considerable path to tread, the concept reflects a leap in AI’s theoretical framework. It represents an ambitious stride to attain artificial intelligence that can operate within the complexities of the real-world, learning, adapting, and evolving just like a human mind. The implications are profound and far-reaching, extending not just to scientific exploration but potentially to every facet of human life profoundly influenced by AI advancements. In essence, BabyAGI is not just an upgrade, but a recalibration, a new catalyst in the sphere of artificial intelligence poised to propel us into a future we are just beginning to grasp.
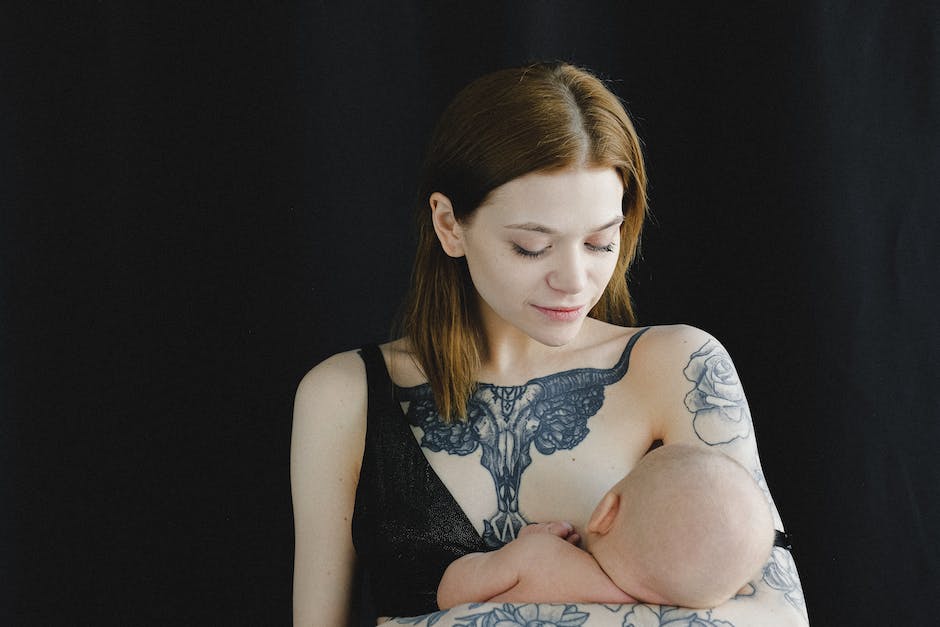
Working Mechanism of BabyAGI
Delving into the Core Mechanism of BabyAGI
As an embodiment of a learning-based model, BabyAGI takes a different tack from the traditional rule-based systems. This paradigm shift leverages the power of unsupervised learning rather than strictly preprogrammed instructions to achieve an advanced level of cognitive functionality in the realm of Artificial Intelligence. Entering the esoteric world of AI, the core mechanism of BabyAGI’s functionality can be encapsulated by its two defining traits: iterative learning and cognitive adaptability.
To begin with, iterative learning is the lynchpin of BabyAGI’s cognitive process. Harnessing the robustness of reinforcement learning and multi-task learning mechanisms, a BabyAGI system embarks on a continual learning journey. It interacts with its environment, collects an expanding repository of experiences, and upon such foundations, refines its knowledge with iterative revisions. This iterative process serves as a stalwart guidepost leading BabyAGI to the attainment of AGI capabilities.
Complementing this process is cognitive adaptability. While iterative learning impels BabyAGI on the journey of information absorption and refinement, cognitive adaptability determines the breadth of its capacity to apply garnered knowledge across a variety of tasks. It enables BabyAGI to accommodate novel problem-solving schemas and transcend the cognitive boundaries delineated by narrow or specialized AI, thereby inching closer to a more nuanced version of AGI.
To facilitate these operations, BabyAGI employs a function approximation model. This mathematical tool serves as the cognitive lens through which BabyAGI perceives and catalogs the world. Function approximators, especially those underpinned by deep learning algorithms, enable BabyAGI to map complex, non-linear relationships within input data. This endows the system with the cognitive lucidity requisite for information interpretation and decision-making.
Simultaneously, the deployment of model-based and model-free methods further enhance BabyAGI’s functional potential. The convergence of these two techniques results in a more balanced operational spectrum. Model-based methods instill the system with the ability to plan a sequence of actions to achieve a target, embedding a strategic element into BabyAGI’s cognitive portfolio. In contrast, model-free methods imbibe the system with the ability to react spontaneously based on previous knowledge, enabling it to tackle immediate problems with tactical acuity, which is vital for dealing with dynamic, real-time challenges.
Working in concert, this cognitive assemblage of iterative learning, cognitive adaptability, function approximation, and a blend of learning methods create a rich tapestry of cognitive capabilities necessary for BabyAGI’s operation. It is through this intricate interplay of components that BabyAGI takes plausible steps towards embodying the vision of AGI, fostering an innovative angle to the field of AI that will reshape our perception of machine capabilities.
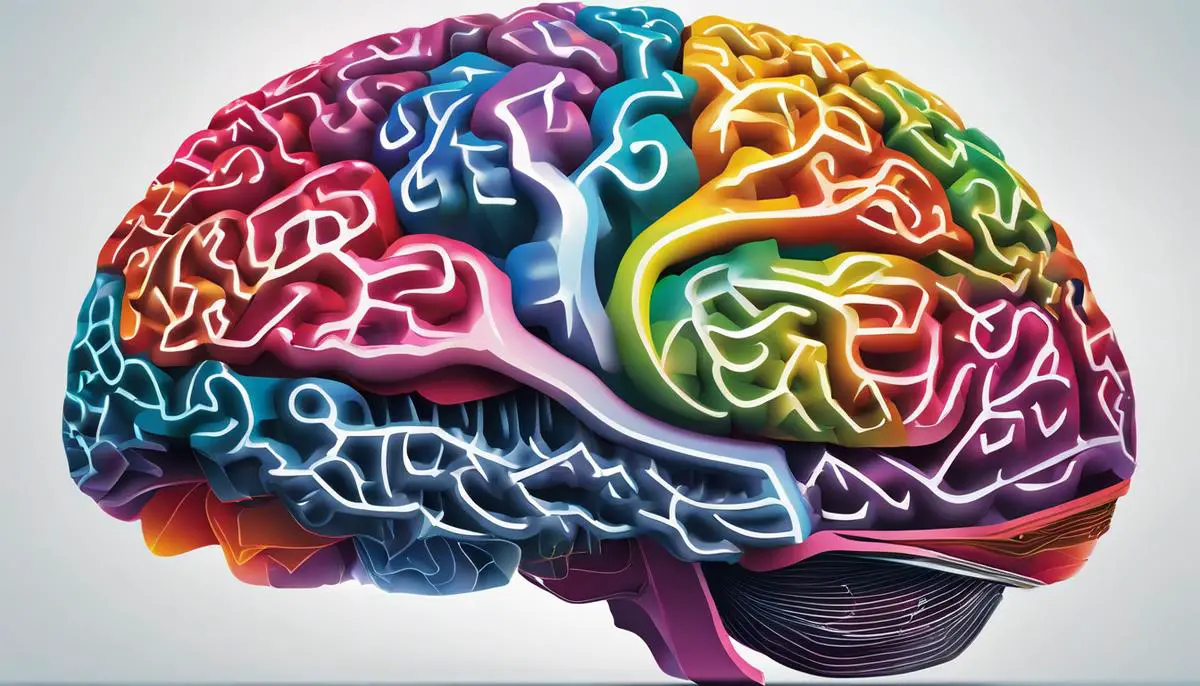
Comparison of BabyAGI with Traditional AI
Therein lies the overarching brilliance of BabyAGI – an innovative blend of iterative learning coupled with the cognitive adaptability of the synthetic intelligence in question. Unlike traditional rule-based AI models that abide strictly by predefined algorithms and lack the ability to learn from experience, BabyAGI leverages the plasticity of learning-based models, allowing it to iterate, learn, and adapt dynamically based on new information and experiences. This cognitive evolution in artificial beings may well be a game-changer in the field of AI.
Iterative learning, in its essence, is inspired by the biological process of learning in humans. With the help of this mechanism, BabyAGI remains in a perpetual state of cognition and learning, refining its dataset and output with each iteration, employing an elegant symphony of trial and error. The iterative nature of BabyAGI’s learning capabilities nicely complements the more traditional supervised and unsupervised learning strategies and acts as a cornerstone for BabyAGI’s strategy to reach a full AGI state.
Bridging the gap between artificial and human intelligence, the trait of cognitive adaptability in BabyAGI presents a fascinating addition to the domain of AI. In human cognitive development, adaptability is a key driver of the ability to solve problems, learn from experiences, and adjust to new or unfamiliar environments. BabyAGI incorporates this human-like cognitive adaptability, equipping itself with a formidable toolbox to navigate and learn from its operational environment, and in doing so, disrupting set AI paradigms.
Moreover, BabyAGI’s cognitive perception and decision-making abilities are significantly enhanced by function approximation models. These models allow BabyAGI to generalize experiences and perceptions from a limited dataset and render correct decisions in unforeseen situations. Just as a human child learns to recognize different breeds of dogs by generalizing their understanding from a few experiences, BabyAGI can also extrapolate from known data to predict unknown outcomes.
Further increasing its operational potential, BabyAGI integrates model-based and model-free methods. While model-based learning allows the system to make predictions and plan by building models of the world, model-free learning focuses on extracting patterns and learning from direct interaction with the environment. This dual-approach significantly heightens BabyAGI’s capability to interpret, predict and interact with its environment.
To sum it all, the power of BabyAGI lies in intricate interplay of iterative learning, cognitive adaptability, function approximation models, and learning methods, enhancing it’s cognitive capabilities and endorsing it as one of the influential catalysts for the advancements in AI.
Unquestionably, BabyAGI may well be the missing link in moving us closer to achieving AGI. With its unique features and outstanding capabilities, it not only advances the field of AI but also changes the narrative from artificial intelligence to artificial cognition, stepping closer to simulating human intelligence in machines. So here lies the testament of what AI is heading for, a potent mixture of cognitive adaptability, iterative learning, functional approximation, and omni-compatible learning methods, all encapsulated in the frame of BabyAGI.
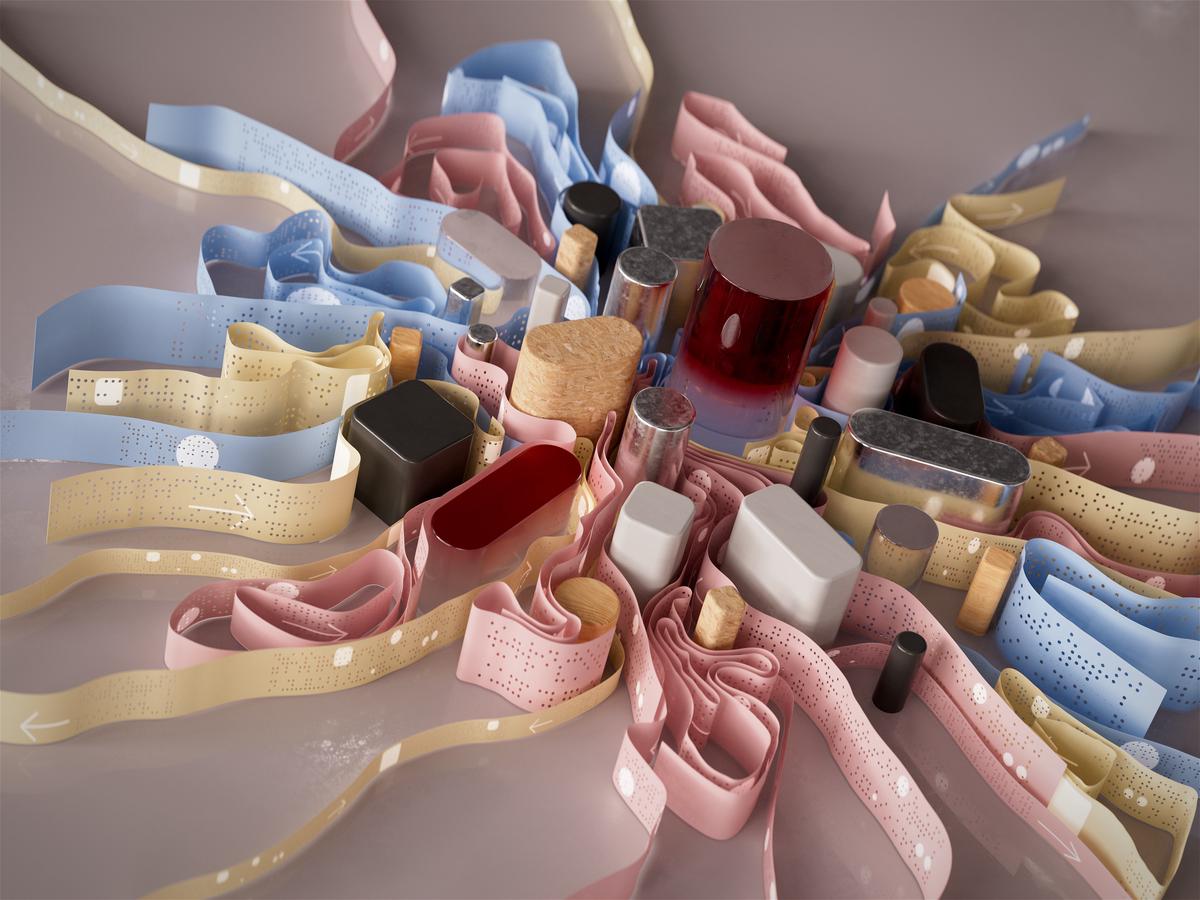
Photo by googledeepmind on Unsplash
The Role of BabyAGI in AI Development
The development of BabyAGI presents a revolutionary leap in AI technology, enriching traditional models of AI with a more dynamic, adaptable approach that posits closer ties with human cognitive capabilities. Its conception draws inspiration from the construct of early childhood development. This analogy holds significant promise in moving beyond the constraints of existing AI models, sparking the next era of cognitive computational science.
One of the extensive wellsprings for BabyAGI’s potential resides in the incorporation of iterative learning – a methodology that stems right from the learning process employed by humans. In essence, BabyAGI’s algorithms embrace the truism that frequent failures, followed by subsequent attempts to rectify those, are a stepping-stone to true learning. This approach aids in the gradual accumulation of knowledge, bridging the gap between simple adaptation and the acquisition of new skills.
Alongside iterative learning, cognitive adaptability assumes a pivotal role in BabyAGI’s conceptual framework. Mirroring the human mind, this facet of BabyAGI provides its machine learning models with the ability to adjust and adapt to different tasks. Through this, BabyAGI moves beyond the rigidity of specialized AI and starts embracing a broader spectrum of problem-solving capabilities, thereby mimicking the fluidity of human cognition.
Function approximation models serve as the backbone of BabyAGI’s cognitive perception and decision-making mechanism. Surpassing the linear arithmetic of conventional algorithms, these models take a lead from its human counterpart, enabling BabyAGI to infer relationships and scrutinize patterns in intricate datasets. This feature not only enhances accuracy but also induces creativity in the decision-making subsystem of BabyAGI.
Accounting for both model-based and model-free methods within its operational sphere, BabyAGI synthesizes and applies knowledge with enhanced finesse. The inclusion of model-based methods promotes a state-value estimation of the environment hence providing a predictive model. In contrast, model-free methods liberate BabyAGI from the limitations of pre-defined models, enabling it to adaptively learn from direct experiences.
The convergence of these features within a singular, comprehensive system forges the enriched cognitive capabilities of BabyAGI. It interweaves iterative learning, cognitive adaptability, function approximation models, and a harmonious blend of learning methods, thus laying the foundation for an AI system that echoes the intuitive fluidity of human cognition.
The prominence of BabyAGI in the odyssey toward AGI cannot be downplayed. Its design, driven by human cognitive processes, propels significant momentum in the journey of simulating human-like intelligence within machines. As a formidable frontrunner in the path toward AGI, BabyAGI anchors foundational development in this field, acquainting us with the immense possibilities that lie ahead.
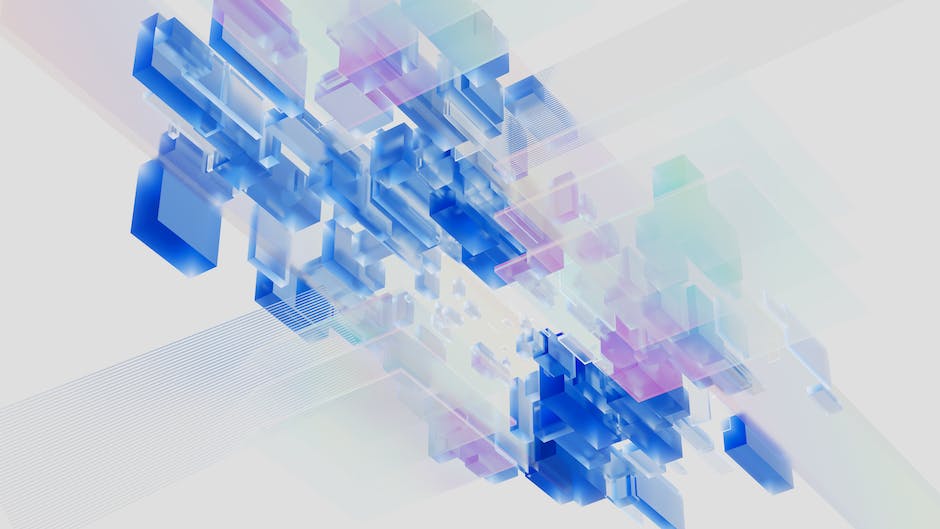
Challenges & Future of BabyAGI
Diving into the Challenges and Future of BabyAGI
The path towards potent Artificial General Intelligence is decorated with numerous challenges and stumbling blocks that the field of AI is persistently grappling with. Undoubtedly, BabyAGI represents a remarkable stride in this great scientific marathon, yet it is crucial to understand the spectrum of hurdles it faces and the implications for future progressions in the field.
Fundamentally, BabyAGI poses daunting computational challenges. Its intricate paradigm involving iterative learning and cognitive adaptability inherently demands powerful computational resources. To mimic the dynamism and complexity of human-like cognitive functions, BabyAGI must efficiently manage an astronomical pool of interconnected multidimensional data points reflecting complex cognitive processes. A constraint in coping with this computational demand can throttle the full potential of BabyAGI.
Moreover, the design and implementation of an effective reward and learning system for BabyAGI present a significant challenge. Similar to child development in humans, BabyAGI aims to refine its learning through feedback and improved iterations. However, providing meaningful, contextually correct feedback for each AI decision or action is time-consuming, resource-intensive, and potentially inconclusive.
An aspect requiring unwavering attention is the ethical and societal implications of BabyAGI development. It hovers around concepts of transparency, accountability and control of AI systems. As BabyAGI inches towards AGI, these ethical considerations balloon in significance, making it imperative to integrate robust transparent mechanisms and ethical guidelines in BabyAGI’s developmental process.
Future Directions for BabyAGI
The trials faced by BabyAGI are substantial but not insoluble. Advancements in computational power, cloud computing, and parallel processing promise to diminish resource constraints, enabling efficient handling of complex multidimensional data.
On the learning front, breakthroughs in reinforcement learning algorithms and the use of pseudo-reward systems provide optimism. They may facilitate the construction of self-regulating data feedback mechanisms, aiding BabyAGI to intuitively fine-tune its cognitive abilities in real-time.
The discourse around ethical and societal considerations is gaining momentum across scientific communities. The increasing focus on explainable AI (XAI) and ethics in AI is valuable. As BabyAGI development gathers steam, embedding these principles from the ground up appears as a promising trend.
BabyAGI undoubtedly embodies a quantum leap in AI. Its paradigm of iterative learning and cognitive adaptability opens up realms of scientific exploration and industrial applications. With advancements converging from all corners of AI research, the orchestration of these elements into the BabyAGI framework could unfold an exciting, unprecedented era in AI evolution. The journey might be laced with challenges, but the destination, a truly intelligent and beneficial AGI, justifies this profound scientific endeavor.
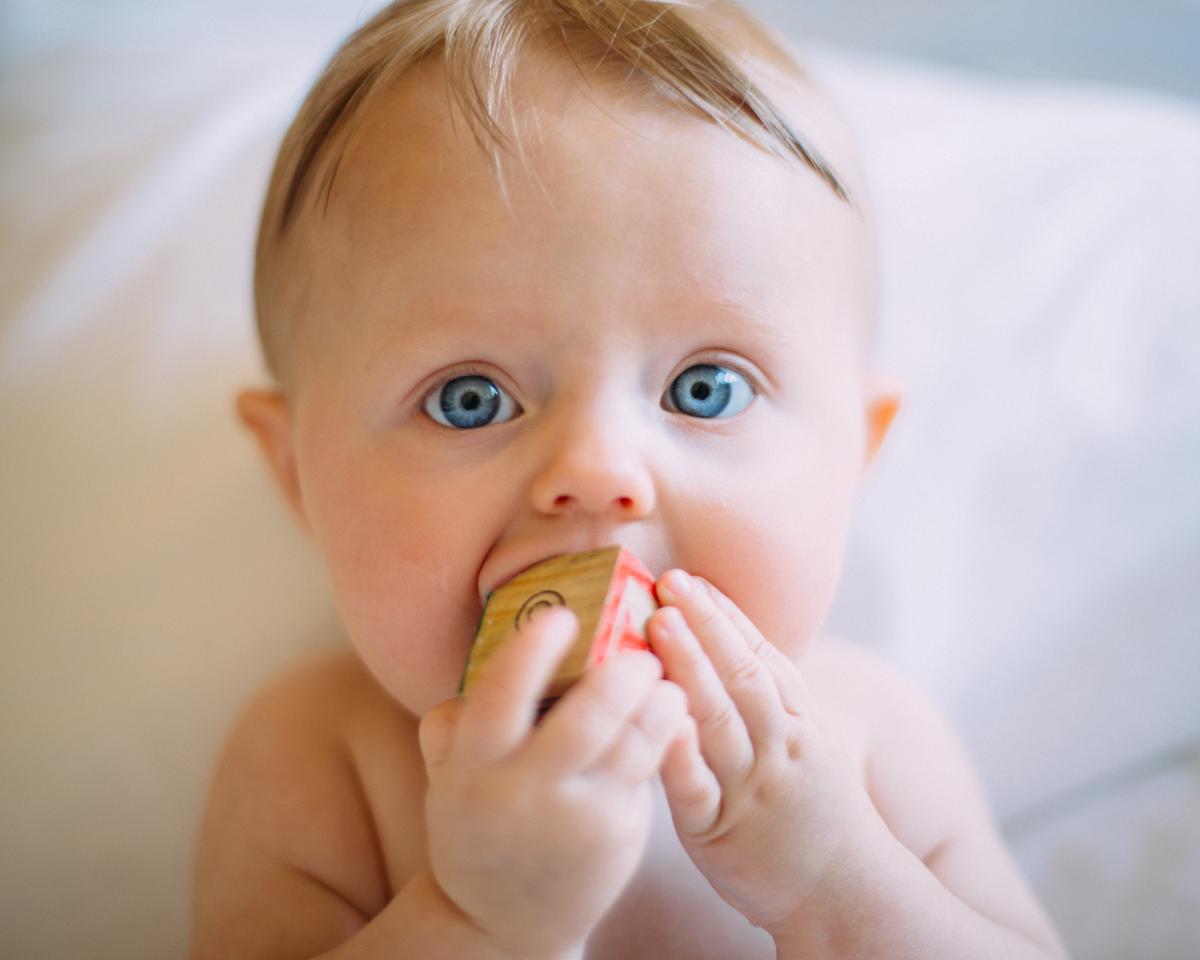
Regardless of the obstacles in the path of BabyAGI, its potential in transforming the world of AI is undeniable. It stands not as a finding, but a profound turning point in the journey of understanding and unraveling the mysteries of cognition, learning, and intelligent decision-making. Perhaps, with time and rigorous exploration, we will see BabyAGI completely revolutionizing AI’s landscape, setting the stage for a new era. An era that promises to marry artificial intelligence to error-reducing, decision-improving continuous learning that could redirect the course of technology and consequently, human civilization.