Object recognition technology in artificial intelligence (AI) has evolved significantly, transforming how machines interpret and interact with the visual world. This exploration aims to shed light on the historical evolution, core principles, and continuous advancements that define object recognition in AI. By understanding these elements, we gain insight into how this technology mirrors human perception and its potential to reshape our interaction with digital environments.
Foundations of Object Recognition in AI
Object Recognition Technology in AI: Unveiling the Core Principles
Object recognition technology in artificial intelligence (AI) marks a significant journey from its rudimentary beginnings to today’s sophisticated systems. At its heart, this technology enables machines to identify and classify objects within images or videos, resembling the way humans perceive the visual world. This exploration provides a window into the historical evolution, fundamental workings, and the continuous improvement that characterizes object recognition in AI.
Historically, the quest for AI to mimic human vision began with basic pattern recognition. Early systems were limited by technology and could only recognize simple shapes or characters. As computational power grew, so did the complexity of recognition tasks AI could undertake. This advancement led to the development of machine learning (ML) algorithms, laying the groundwork for modern object recognition.
Central to understanding object recognition in AI is recognizing how these systems interpret visual data. Unlike humans, who can easily distinguish objects from a glance, AI systems digest an image as an array of pixels. The challenge lies in teaching the AI to recognize patterns within these pixels that correspond to specific objects. This process involves breaking down images into features, such as edges, textures, or colors, which serve as identifiable markers for different objects.
The advent of deep learning, particularly convolutional neural networks (CNNs), has been pivotal in enhancing object recognition. CNNs simulate how the human brain operates to some extent, using layers of artificial neurons to process and interpret visual data. Each layer focuses on different aspects of the image, starting from basic features to more complex representations, enabling the system to eventually identify objects with impressive accuracy.
A cornerstone of AI’s object recognition technology is its ability to learn from new information. Through exposure to vast amounts of visual data, these systems refine their understanding and improve their ability to distinguish between objects. This self-improving capability underscores the dynamic nature of AI, which can adapt and evolve its recognition accuracy over time.
Moreover, object recognition technology is enriched by considering multiple perspectives. Just as a cup viewed from the side presents a different shape than one viewed from above, AI systems must learn to recognize objects from various angles and under different lighting conditions. This flexibility adds a layer of complexity but is crucial for achieving user-friendly and reliable recognition in everyday scenarios.
As object recognition technology continues to evolve, its implications stretch across a myriad of applications, from autonomous vehicles navigating through the streets to smartphones unlocking with facial recognition. By delving into the historical milestones, understanding the mechanism of visual data interpretation, and appreciating the constant learning nature of these systems, one gains a comprehensive insight into the foundational principles that guide object recognition technology in AI.
This exploration offers a glimpse into a field that stands at the intersection of technology and human-like perception, providing a clear window into how machines learn to see and understand our world.
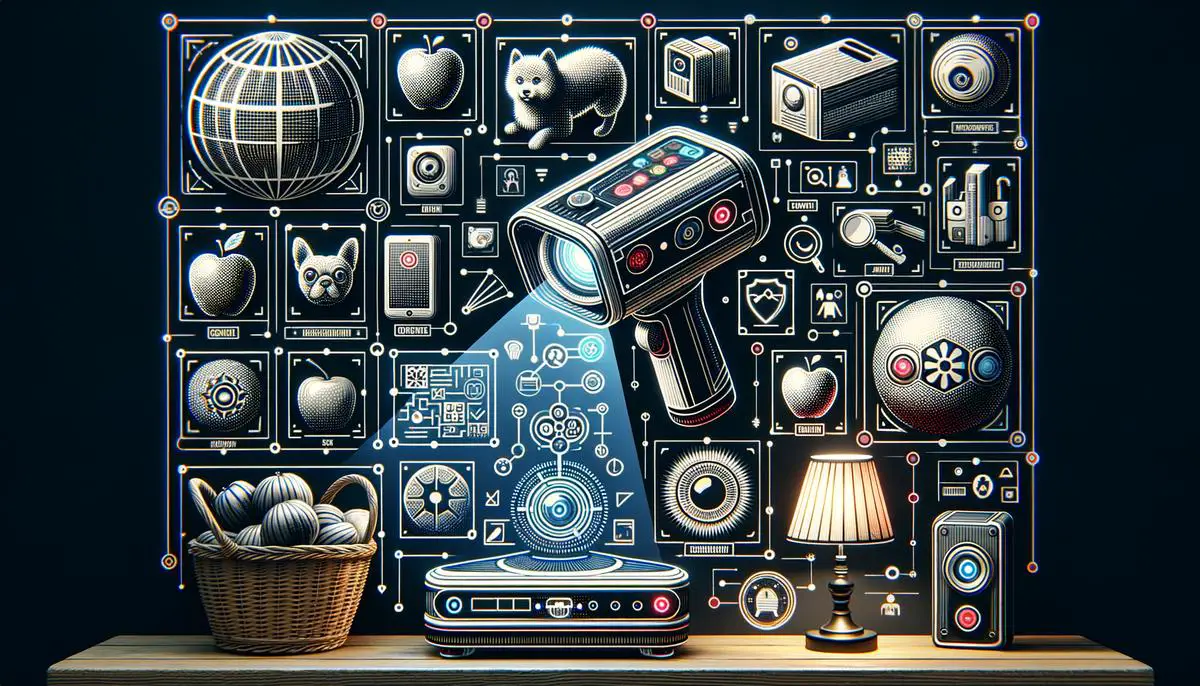
Key Technologies Behind Object Recognition
Advancements in the field of artificial intelligence (AI) have significantly propelled object recognition systems into newfound realms of proficiency. Two pivotal technologies at the heart of these systems are machine learning and deep learning algorithms, which orchestrate the most sophisticated object recognition capabilities seen today. Here, we hone in on convolutional neural networks (CNNs) and the instrumental role GPUs play in realizing AI’s potential in recognizing and understanding the visual world around us.
Convolutional Neural Networks (CNNs) stand as a cornerstone technology, especially tailored for the demands of image recognition tasks. Structurally, CNNs mimic the human visual cortex’s hierarchical pattern, where neurons respond to specific stimuli in the visual field. This analogy extends to how CNNs discern and process visual data, comprising successive layers designed to filter and compile essential aspects of images. Each layer in a CNN focuses on different attributes: while the initial layers might capture basic edges and textures, deeper layers synthesize these features to recognize complex objects.
In action, CNNs require vast datasets for training, where they undergo the process of learning from thousands, if not millions, of images. Through this extensive exposure, they continually adjust and refine their internal parameters for better accuracy in object recognition tasks. It’s the fusion of depth and vast training that furnishes CNNs with unparalleled capabilities in detecting and identifying a broad spectrum of objects within an image.
Yet, the monumental task of processing such immense datasets and executing these complex algorithms necessitates another critical technology: Graphics Processing Units (GPUs). Originally designed to accelerate image and video rendering, GPUs possess a multitude of cores capable of performing parallel computations much more efficiently than their Central Processing Unit (CPU) counterparts. This characteristic makes them exceptionally apt for the rigorous calculations involved in deep learning tasks.
GPUs thus serve as the engine room for modern object recognition systems, enabling the real-time data processing essential for applications ranging from autonomous vehicles navigating bustling streets to smartphones identifying faces in cluttered scenes. They work in tandem with the meticulous structure of CNNs, speeding up the enormous number of computations required for identifying and recognizing objects in images.
In essence, machine learning and deep learning, through the medium of convolutional neural networks, paired with the computational might of GPUs, form the backbone of current object recognition technologies. This strategic combination of AI algorithms and high-powered processing not only facilitates a deeper understanding of our visual world but also propels us towards further realms of what artificial intelligence can accomplish in terms of object detection and recognition. Through ongoing developments in these areas, we can anticipate even more sophisticated applications that will continue to blur the boundaries between AI-generated perceptions and human vision.
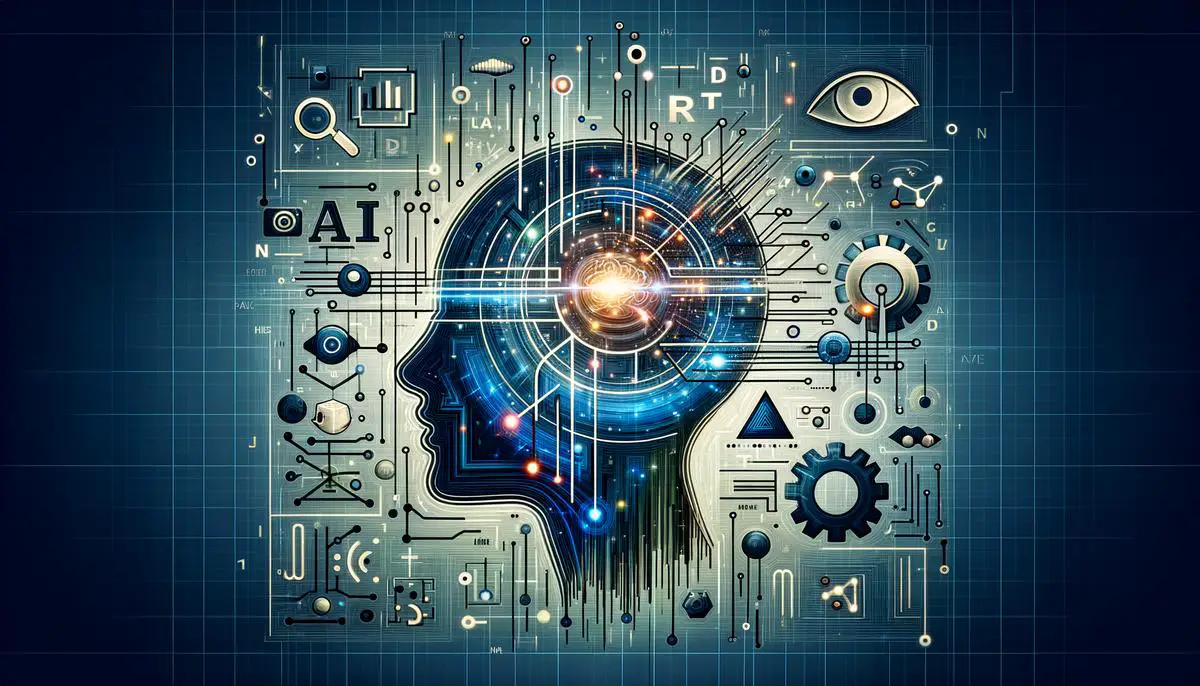
Applications of Object Recognition
Object recognition technology finds its application spanning a diverse array of industries, demonstrating its multifaceted importance and the breadth of its functionalities. From enhancing security measures to propelling the healthcare sector into a new era of diagnosis, the versatility of object recognition technology is clear. Delving into the specifics, we see its profound impact across various sectors in equally substantial ways.
In the realm of security and surveillance, facial recognition systems stand at the forefront of object recognition applications. These systems can instantly identify individuals among crowds, comparing faces against vast databases with remarkable accuracy. This capability not only bolsters security protocols for both public and private spaces but also aids law enforcement in identifying suspects or missing persons more swiftly than ever before.
The healthcare sector benefits from object recognition through advances in medical imaging analysis. This technology allows for the precise and faster identification of abnormalities in images like X-rays, MRIs, and CT scans, contributing to earlier and more accurate diagnoses. Recognizing patterns in medical imaging that may elude the human eye, this application of object recognition supports medical professionals in crafting effective treatment plans for their patients.
In the automotive industry, autonomous vehicles utilize object recognition to navigate roads with increasing autonomy. Through processing live data from cameras and sensors, these vehicles can identify and respond to objects, pedestrians, and other vehicles in real-time, a critical development for ensuring the safety and feasibility of self-driving cars.
Object recognition technology is revolutionizing the retail world as well, particularly through automated checkout systems. By identifying products without the need for barcodes and processing transactions seamlessly, these systems offer a glimpse into the future of shopping. They reduce wait times, minimize errors, and enhance the customer experience, showcasing the potential for widespread adoption across the retail sector.
Drawing upon these examples, it becomes evident that object recognition technology is not merely an auxiliary tool but a cornerstone development that’s reshaping industries. Through its myriad applications, from improving security to pioneering advancements in various fields, the influence of object recognition is steeped in both innovation and practicality. Its ability to transform core operations within diverse sectors underscores not only the present achievements but also the untapped potential for future innovations within and beyond these industries.
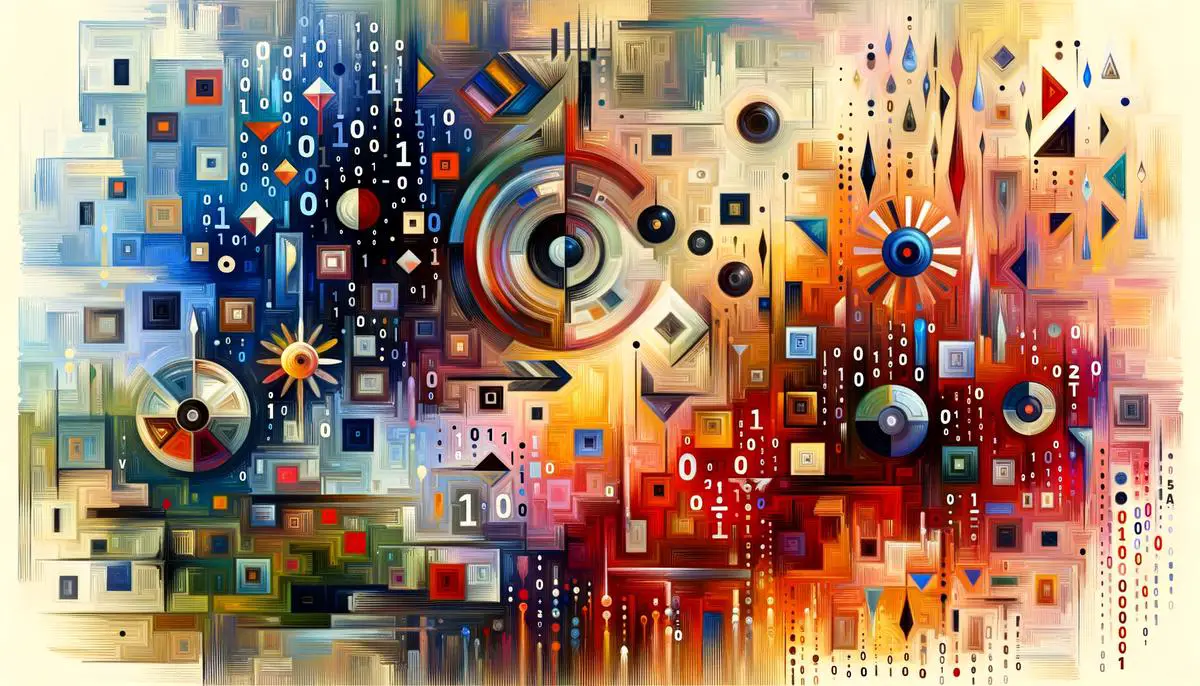
Challenges and Limitations
Object recognition technology has made tremendous strides, enhancing various sectors from healthcare to security. However, it faces several challenges and limitations that must be addressed for it to reach its full potential. Among the primary concerns are accuracy issues, privacy worries, and the ethical implications linked to surveillance.
Accuracy in object recognition is critical, yet achieving it remains a challenge. Even with advanced machine learning algorithms, these systems can struggle to identify objects under certain conditions. For example, poor or varied lighting can significantly affect a system’s ability to recognize an object. This complication arises because different lighting conditions alter the appearance of objects, making them look vastly different from the examples the system was trained on.
Moreover, recognizing objects from various angles poses another significant hurdle. When an object is not positioned in the same way as in the training data, the system might fail to recognize it. This limitation closely ties in with the challenge of identifying partially obscured objects. If an object is not fully visible, current technology often struggles to recognize it correctly, affecting the accuracy and reliability of these systems across real-world applications.
Beyond the technical challenges, privacy concerns stand as a significant barrier to the wider adoption of object recognition technology. With the capability to track and recognize individuals’ faces or personal belongings, there arises the risk of intrusive surveillance. This leads to heated debates on the need for strict regulations and guidelines to ensure that privacy is not compromised under the guise of technological advancement.
The ethical implications of widespread surveillance through object recognition technology cannot be overstated. As these systems become more integrated into daily life, the potential for misuse escalates. The idea of being constantly monitored raises alarms about the erosion of personal freedoms and privacy. Moreover, the potential of biased data further complicates matters, as inaccuracies in object recognition could disproportionately affect certain groups, leading to unfair treatment or discrimination.
These challenges underscore the urgency for developers and policymakers to address not only the technical limitations but also the ethical and privacy concerns surrounding object recognition technologies. As advancements continue, striking a balance between innovation and respecting individual rights will be crucial for society to benefit fully from these technologies without overarching negative repercussions.
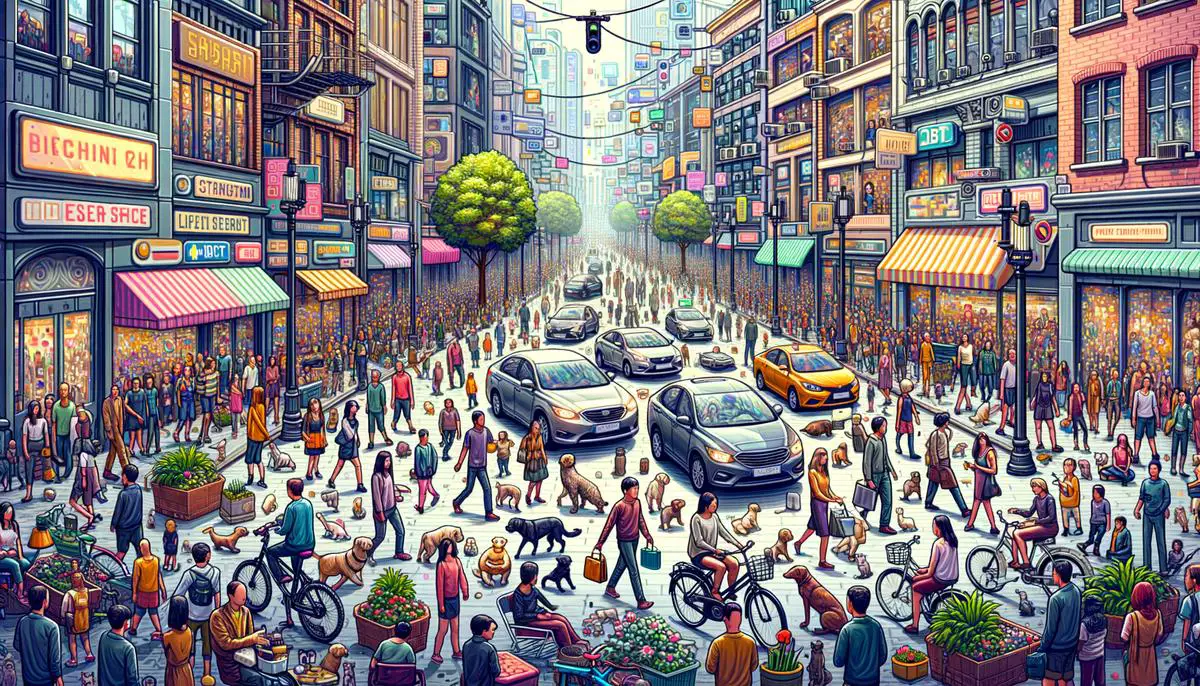
The Future of Object Recognition
Moving forward, as we unravel the trajectory of future developments in object recognition technology, it’s essential to investigate how rising efficiencies in algorithms, pairing with cutting-edge technologies such as augmented reality (AR), and the ever-expanding accurate object recognition capabilities, are set to reshape societal norms. This exploration is not merely about envisaging the next generation of technological breakthroughs but understanding the broader implications and possibilities they usher in for diverse applications, from everyday conveniences to complex problem-solving in industries.
In the realm of algorithm efficiency, we stand on the brink of witnessing revolutionary strides. The core of this evolution leans heavily on refining the algorithms’ ability to parse and process visual data more swiftly and accurately. The future could see algorithms that learn and adapt at an unprecedented pace, reducing the computational load and thereby enabling more portable and accessible applications of object recognition technology.
This segue into the pairing of AI with emergent technologies like AR offers a tantalizing glimpse into a future where the digital and physical worlds converge seamlessly. Imagine AR glasses that not only project information onto the real world but do so with an understanding of the objects in their wearer’s vicinity. Such integration would elevate user interactions with both digital content and the real environment, enabling a symbiotic relationship between technology and reality.
Yet, the crux of future advancements is not solely grounded in technical prowess but also in the societal impacts of pervasive and accurate object recognition. As this technology filters more into the fabric of daily life, its applications could extend far beyond current uses. From enhancing the shopping experience through virtual fitting rooms to revolutionary changes in elder care through constant environmental monitoring, the implications are profound.
Simultaneously, ongoing research aimed at conquering current limitations is pivotal. Challenges such as interpreting complex scenes with numerous overlapping objects or recognizing items in variable lighting conditions are actively being addressed by researchers. Their work promises a future where object recognition technology could offer near-human levels of perception, if not surpass it.
Moreover, by expanding the technology’s applications, new frontiers are being charted. Agriculturists could benefit from drone-assisted crop analysis for detecting pests or disease symptoms, whereas in disaster management, quickly identifying structural damages or hazards could save lives. Such developments hinge on making object recognition more adaptable, rugged, and capable of functioning in diverse and challenging environments.
As we look to the horizon, it’s clear that object recognition technology is primed for a quantum leap forward. Yet, its trajectory will need to balance on the fulcrum of innovation and ethical consideration, ensuring that as our capabilities expand, they do so in harmony with societal values and individual rights. The discussions today around privacy, bias correction in AI, and ethical deployment will shape not just the future of object recognition technology but how it’s interwoven with the very fabric of our daily lives.
Therefore, as we venture into this brave new world of object recognition, it’s essential to carry forward a spirit of curiosity coupled with responsibility. The infinite possibilities must be navigated thoughtfully, ensuring that while we break boundaries with technological advancements, we also build a future that enhances human experiences while safeguarding fundamental rights and dignity.
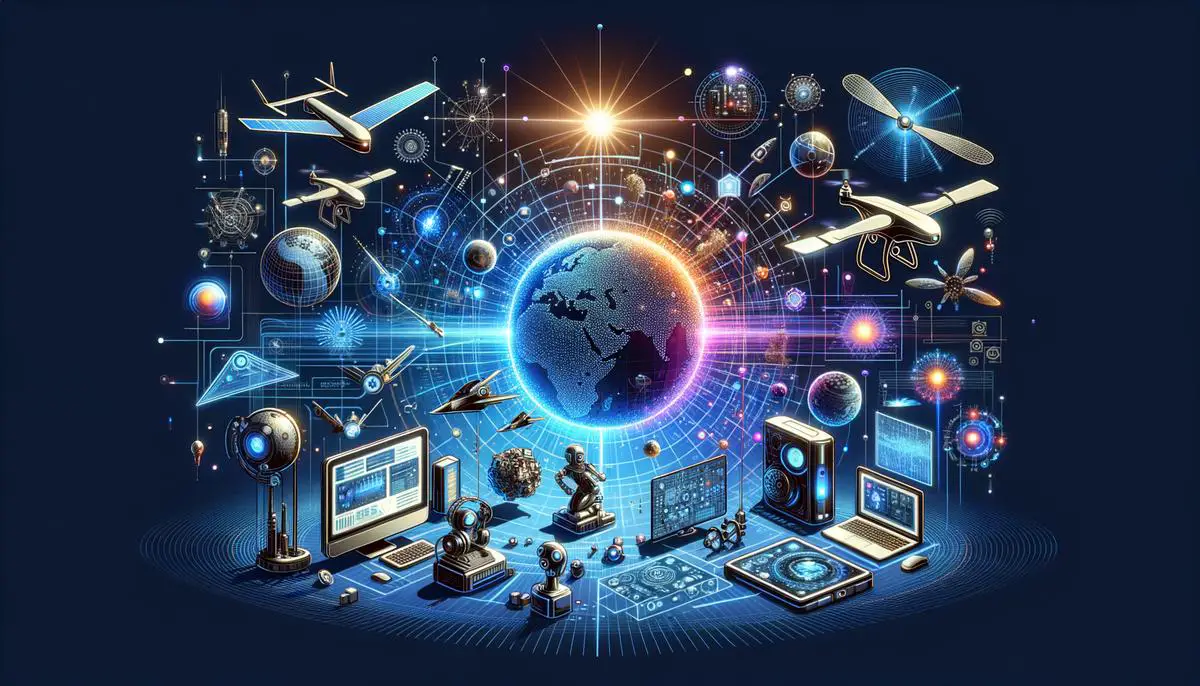
As we explore the vast landscape of object recognition technology, it becomes evident that this field stands at a critical intersection of innovation and practical application. The advancements in AI not only push the boundaries of what machines can achieve but also open new avenues for enhancing human experiences across various sectors. Embracing these changes requires a thoughtful approach to ensure that technological progress aligns with ethical standards and respects individual rights. As we move forward, the future of object recognition promises not just a leap in technological capabilities but also an opportunity to redefine our relationship with the digital world.