Image segmentation is a powerful tool in the realm of artificial intelligence, enabling computers to interpret and understand images with remarkable precision. This process, essential for various applications from self-driving cars to augmented reality, relies on cutting-edge techniques and innovative algorithms. As we explore the intricacies of image segmentation, we’ll uncover how it’s transforming our interaction with technology and opening up new possibilities across different fields.
Understanding Image Segmentation
In the expanding universe of artificial intelligence (AI), image segmentation stands out as a critical process that helps computers see and understand images similarly to how we do. Imagine trying to piece together a puzzle; image segmentation works in much the same way by breaking down an image into its constituent parts or pixels. This breakdown is not just a random division; instead, it’s a precise separation aimed at improving the machine’s ability to recognize, categorize, and work with images on a much more detailed level.
Let’s dig into the nuts and bolts of it.
Fundamentally, when we talk about image segmentation, we’re referring to the process where an AI system takes a digital image and splits it into multiple segments or pieces. These pieces could be parts like distinct objects within the image, borders, or even the background. The idea is to simplify or change the representation of an image into something that’s easier to analyze and understand. It’s somewhat akin to dividing a giant block of text into paragraphs—suddenly, it becomes a lot easier to navigate and make sense of.
Why does this matter? In applications where understanding context and details within an image are crucial—think of self-driving cars needing to distinguish between a pedestrian and a lamppost—image segmentation is invaluable. It enables tasks such as object detection, which allows a system to recognize objects within images; image classification, where the system classifies what it sees in the image; and scene understanding, which involves comprehending what’s happening in an image. All these tasks get a significant boost from being able to work with more finely segmented images.
Consider how a self-driving car processes the data it sees. It doesn’t just see a road with cars and people; through image segmentation, its AI breaks down the scene into pedestrians, vehicles, traffic lights, and so forth. This granularity helps the AI make more informed decisions like when to stop, speed up, or swerve.
In practice, there are a few techniques and methods involved in image segmentation. Without diving too deep into technical jargon, these can range from straightforward approaches like setting a threshold for color contrasts, to more complex ones that involve teaching the AI system through examples (a method known as supervised learning). The choice of method often depends on the specific task at hand and the complexity of the images being analyzed.
Engaging with image segmentation, AI systems become more adept at analyzing our world in its visual complexity, opening doors to innovations across various fields—from healthcare diagnostics to enhancing online shopping experiences. As we continuously refine these technologies, computers get closer to understanding our visual world with clarity and precision that rival human perception.
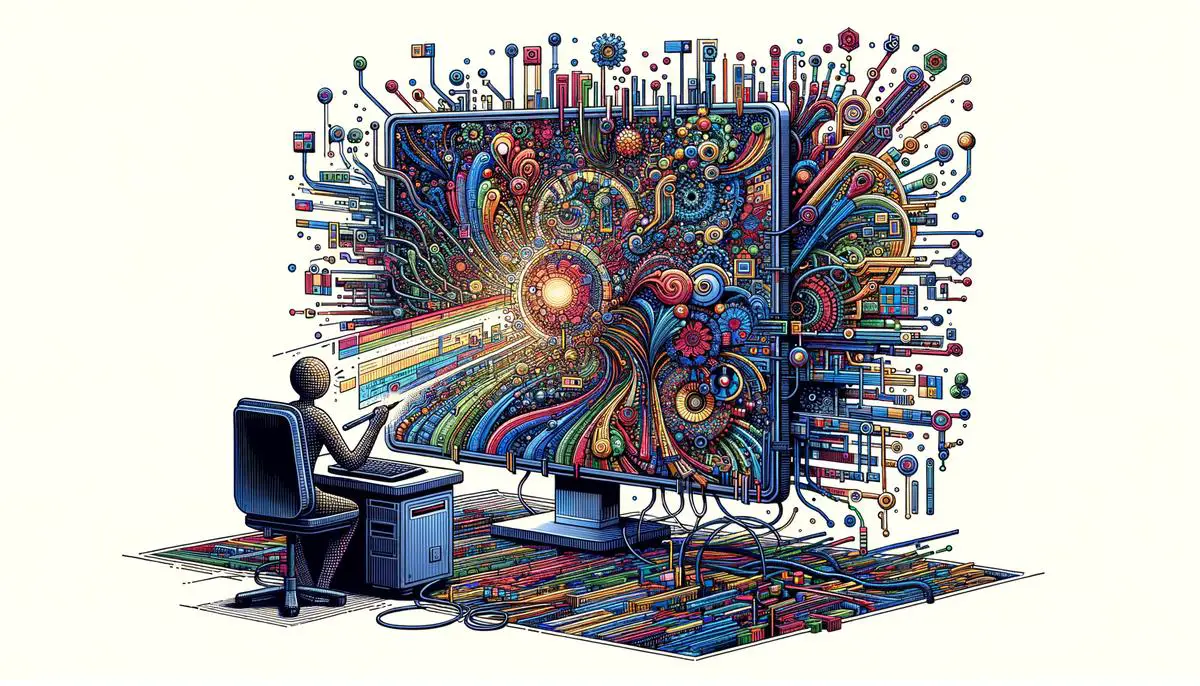
Meta AI’s Approach to Image Segmentation
Meta AI’s strategy for tackling image segmentation merges the power of cutting-edge computing technologies with innovative algorithms, setting a new standard for precision and performance in this field. Central to its approach are machine learning models that have been meticulously developed to break down and understand complex images at an unprecedented scale.
For starters, Meta AI harnesses the prowess of deep learning, a subset of machine learning where neural networks — vast, interconnected web-like structures — mimic the learning process of the human brain. These networks go through layers of training, allowing them to recognize intricate patterns and nuances in the data they process. Specifically, Convolutional Neural Networks (CNNs) are at the heart of Meta’s image segmentation thrust. CNNs are particularly adept at processing pixel data, making them ideal for dissecting and analyzing images.
Improving upon traditional methods, Meta AI’s algorithms are designed to be self-improving. They do this by constantly learning from vast amounts of tagged data — a process known as supervised learning. However, what sets Meta apart is its leveraging of semi-supervised and unsupervised learning techniques. These methods require less human-labeled data, a significant advantage since tagging data can be resource-intensive. Instead, the system learns to recognize patterns and categorize data autonomously, improving efficiency.
Edge computing plays a pivotal role in Meta’s methodology. By processing data closer to where it’s collected (like on a smartphone or a local server), decisions can be made faster, reducing latency. This is crucial for applications requiring real-time processing, like augmented reality experiences or instant video modifications.
Meta’s approach differs in how it balances the computational load. Traditional image segmentation techniques often rely heavily on cloud computing resources, which can create bottlenecks and slow down processing times. Meta AI uses more advanced on-device processing, which not only speeds up the image segmentation process but also addresses privacy concerns by minimizing the amount of data that needs to be sent over the network.
Incorporating advances in artificial intelligence research, Meta AI has developed unique segmentation models that are capable of handling the complexities of real-world imagery. These models are trained to distinguish between myriad object types in various conditions — be it different lighting, angles, or partially obscured objects. For instance, while traditional methods might struggle with overlapping objects or varying textures, Meta’s algorithms excel at parsing these complexities with greater accuracy.
Additionally, Meta AI focuses on scalability. With the ever-increasing volume of images generated daily on platforms like Instagram and Facebook, scalability is key. Their algorithms are designed to maintain accuracy and efficiency even as they scale over vast networks and massive datasets.
Lastly, Meta AI is pioneering in its collaborative approach. Recognizing the value of shared knowledge in pushing the boundaries of what’s possible, Meta actively participates in the broader AI research community. By publishing their findings and releasing open-source tools, they contribute to accelerating advancements not just within their projects, but across the industry.
Through a blend of deep learning, innovative processing strategies, and a community-driven approach, Meta AI is redefining the landscape of image segmentation. Their methods not only enhance the performance and application of AI in this area but also pave the way for future innovations that will further enrich our interaction with digital imagery.
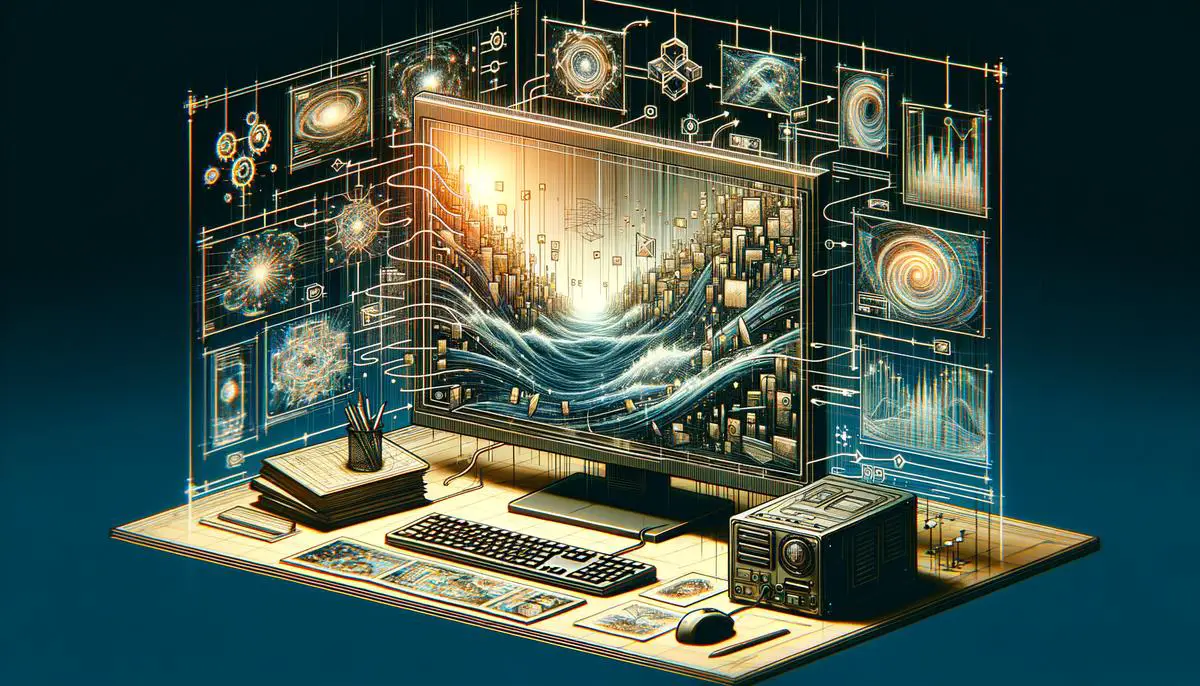
Applications of Image Segmentation in Meta Platforms
Meta, formerly known as Facebook, Inc., has made significant strides in integrating image segmentation across its suite of applications and services, leveraging this technology to provide a more engaging and personalized user experience on Facebook, Instagram, and Oculus. Here’s a breakdown of how image segmentation finds utility in Meta’s ecosystem, contributing to advancements in augmented reality (AR), content moderation, and targeted advertising, among other areas.
In the realm of augmented reality, particularly on Instagram and Facebook, image segmentation is the unsung hero behind some of the most captivating AR filters and effects that users interact with daily. When you apply a cute dog ear filter or immerse yourself in a virtual landscape, image segmentation algorithms are working diligently behind the scenes. These algorithms dissect the image, distinguishing your face from the surrounding environment to overlay the digital effects accurately. This ensures that the ears sit perfectly atop your head or that the virtual world doesn’t awkwardly overlay on top of you, enhancing realism in these AR experiences.
Content moderation is another critical area where image segmentation proves indispensable within Meta’s platforms. Given the vast amount of visual content uploaded every minute, manually monitoring these uploads for compliance with community standards is an impossible task. Image segmentation steps in here, helping to automatically identify and categorize content that might be problematic, such as graphic violence or explicit material, by isolating specific elements within images. This allows for a more speedy review process, ensuring that Meta’s platforms remain safe and welcoming for users of all ages.
Targeted advertising, a cornerstone of Meta’s revenue stream, also benefits immensely from advancements in image segmentation technology. By analyzing images shared by users on Facebook and Instagram, image segmentation helps paint a clearer picture of an individual’s interests and preferences. For instance, if you frequently post pictures of hiking trails or outdoor adventures, image segmentation can identify these themes, enabling advertisers to serve you ads that are more relevant to your interests, such as camping gear or travel destinations. This not only increases the efficacy of ads but also enhances user satisfaction by minimizing exposure to irrelevant ads.
Finally, in the ever-evolving domain of virtual reality (VR), particularly with Oculus devices, image segmentation plays a pivotal role. It facilitates the creation of immersive VR environments that demand precise interaction between the virtual and real worlds. Image segmentation algorithms help distinguish between different objects and users’ limbs in real-time, allowing for more natural and intuitive interaction with VR content. This not only improves the overall user experience but also broadens the possibilities for application development within these virtual spaces.
To sum up, image segmentation is a versatile technology that underpins many features and functionalities across Meta’s platforms. From enhancing AR experiences with realistic filters to ensuring safer online communities through effective content moderation and enabling more personalized advertising experiences, image segmentation’s role is both profound and multifaceted. As Meta continues to push the boundaries of what’s possible within social media and VR, image segmentation’s importance is only set to grow, crafting more engaging and personalized digital experiences for users worldwide.
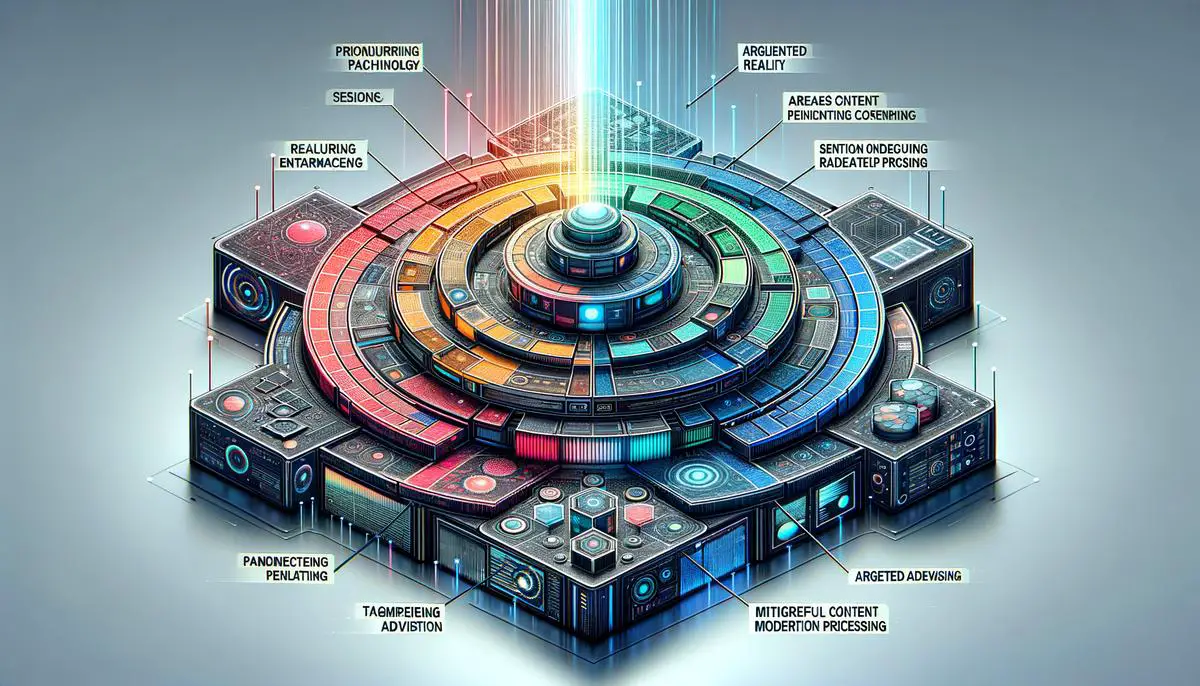
Challenges and Solutions in Image Segmentation
Moving dive into the myriad challenges that Meta AI confronts in the realm of image segmentation further spells out just how intricate the process gets beyond its apparent benefits and applications. One significant hurdle sticks out: data privacy. In an age where personal images are frequently uploaded to platforms like Instagram and Facebook, ensuring users’ privacy while training AI models requires a tightrope walk. Meta has responded by deploying on-device processing methods, suggesting a stride towards handling data sensitively, without extra compromising user privacy.
Another challenge that cannot be overlooked concerns the computational resource demands inherent in processing vast quantities of images. Image segmentation, particularly with high-resolution content, demands a significant amount of computational power, which can strain even the most robust systems. Meta AI has taken bold steps toward mitigating this by refining algorithm efficiency and leveraging edge computing technology. This allows a decent chunk of the processing workload to be carried out on local devices, thereby reducing the demand on central servers and cutting down on the latency that users might experience.
The elephant in the room, however, might just be the handling of diverse and dynamic visual content. Given how users’ photos and videos vary widely in quality, context, and subject matter, training AI models to accurately perform image segmentation across such a broad spectrum proves challenging. Meta AI has tackled this by implementing sophisticated neural networks and continually enriching their training datasets with a broad range of images. By embracing an approach that favors continuous learning and adaptation, Meta AI aims to enhance its models’ ability to discern and segment images more accurately over time, regardless of the diversity in the visual content it encounters.
In round-ups, while the journey embarks Meta AI through an obstacle-laden path in mastering image segmentation, the strategies woven into their fabric reflect a committed resolve towards overcoming these challenges. Through a blend of respecting user privacy, optimizing computational resources, and handling an eclectic mix of images, Meta AI is slowly but surely enhancing its prowess in machine vision. This ongoing endeavor does not only push the boundaries of what’s possible within the realms of AI and AR but also hints at an ever-evolving landscape of digital interactions awaiting on the horizon.
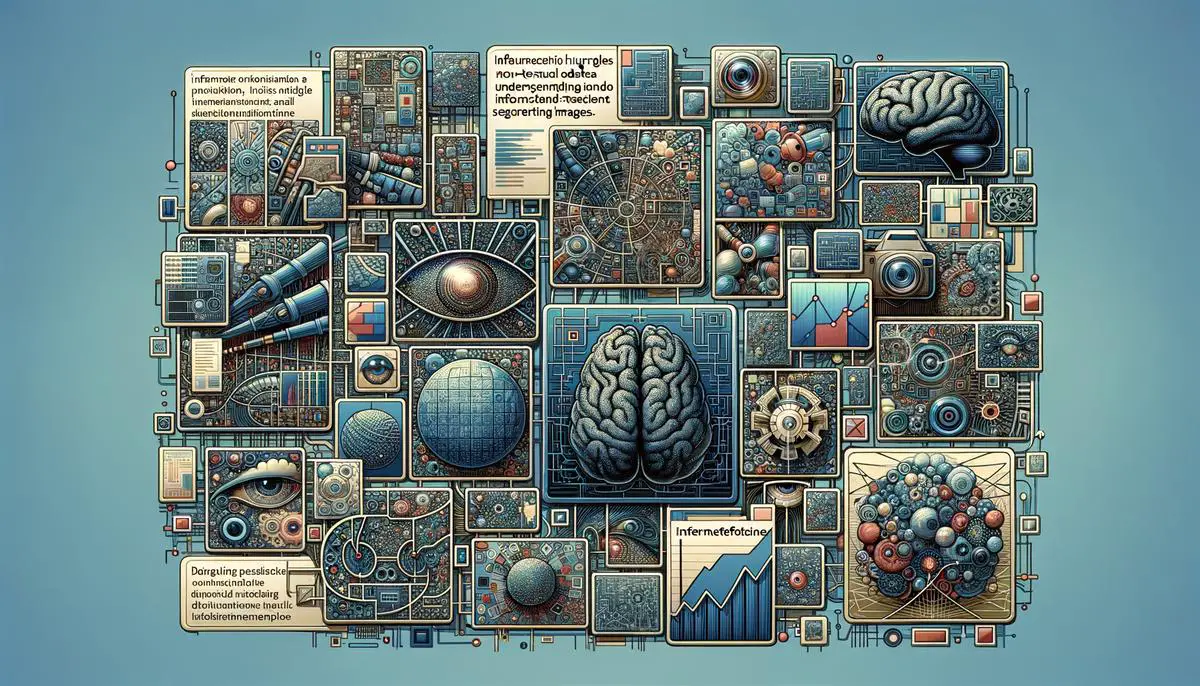
Future of Image Segmentation in AI
Looking forward, the trajectory of image segmentation is bound only by the limitations of current technology and our ability to innovate beyond them. Emerging AI techniques, particularly within the realms of deep learning and neural networks, beckon a future where image segmentation could experience leaps in efficiency and functionality, significantly impacting both Meta’s array of services and the broader technological environment.
A prime area for potential advancement lies in the autonomous improvement of algorithms. As these systems gain the ability to not just learn but to iteratively refine their learning processes, we may see a shift towards more adaptive, resilient image segmentation methods. This could lead to algorithms that more accurately interpret nuances in images, like differentiating between subtle shifts in lighting or discerning complex patterns, without human intervention for recalibration.
Deep learning’s potential to unlock even more sophisticated neural networks hints at a future where image segmentation can process images with near-human levels of interpretation. Enhanced computational models might predict and incorporate context, imbuing machines with a sort of ‘intuition’ about what they’re ‘viewing.’ This leap would translate into exceptionally detailed, accurately segmented images invaluable for applications requiring precision, from medical diagnostics to autonomous vehicle navigation.
Another consequential development might emerge from refining synergies between AI and quantum computing. This partnership could drastically reduce the time required for data processing, enabling real-time image segmentation across massive datasets impossible with current technologies. Such speed and efficiency not only benefit companies like Meta in improving user experience but also hold profound implications for fields requiring rapid data analysis, such as disaster response or security surveillance.
The social implications of these advancements also cannot be understated. As image segmentation becomes more integral to digital experiences, ethical considerations surrounding privacy and surveillance become paramount. Here, we might anticipate innovations centered around secure, privacy-preserving technologies that ensure data protection while still leveraging the benefits of advanced image segmentation.
Moreover, democratizing access to these powerful tools will likely become a focal point. As the capabilities of image segmentation evolve, so does the necessity for accessible interfaces that empower users across various disciplines to harness this technology without needing specialized training in AI or computer science.
In summary, while the path forward for image segmentation holds numerous technical challenges, it also brims with opportunities for significant advancement. From refinements in algorithmic self-improvement and neural network sophistication to groundbreaking integrations with quantum computing, the future promises enhanced efficacy, speed, and application of image segmentation. Coupled with mindful consideration of the social and ethical implications of these technologies, the future of image segmentation stands to not just augment but in some cases, transform our digital and physical realities.
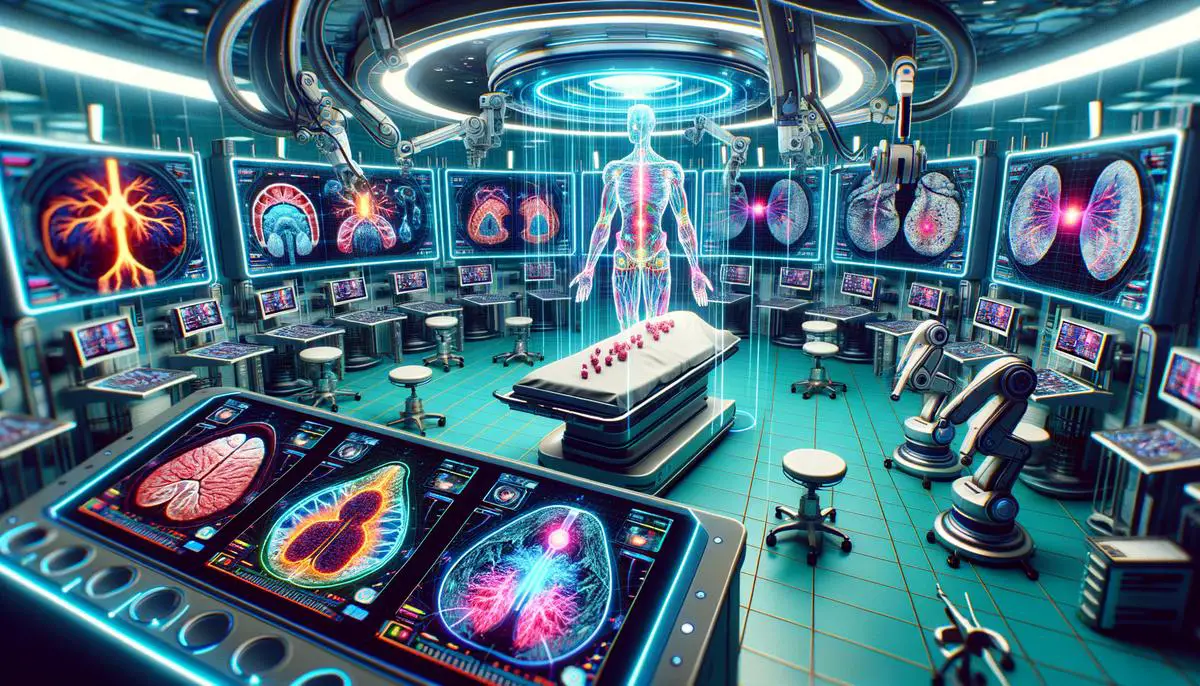
As we stand on the brink of new advancements in image segmentation, it’s clear that this technology holds the key to unlocking unprecedented levels of detail and understanding in digital imagery. The future promises not only more sophisticated methods but also broader applications that could redefine our digital experiences. With ongoing research and development, image segmentation is poised to enhance our world in ways we are just beginning to imagine, making every pixel count towards a more connected and visually rich future.